Search engine optimization (SEO) has undergone a massive transformation, evolving from a keyword-centric strategy to a more sophisticated, context-driven approach. The introduction of semantic search and entity-based optimization has reshaped how search engines interpret and rank web content. This shift means that Google and other search engines prioritize understanding relationships between entities rather than merely matching keywords.
In a recent Entity Hour episode hosted by Terry Van Horne and Bill Slawski, guest Dixon Jones of InLinks explored the implications of this new SEO paradigm. This article delves into the core concepts discussed during the conversation, including Google’s transition from keyword-based search to entity recognition, the role of schema markup, and the future of search engine optimization.
What is semantic SEO?
Semantic SEO refers to an approach that goes beyond keyword matching to understand user intent and the contextual meaning of queries. Rather than simply looking at text strings, Google interprets search queries as concepts or entities and seeks to provide the most relevant information.
Bill Slawski explained that search engines now focus on “real-world objects,” meaning they analyze and index facts about people, places, events, and businesses rather than just words on a page. This approach allows Google to connect different pieces of information and rank search results based on relationships rather than simple keyword frequency.
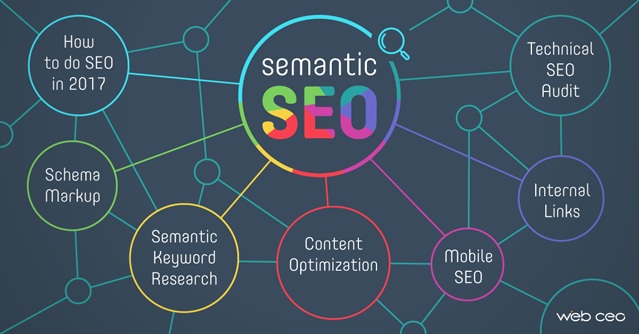
Google’s shift from crawling webpages to crawling entities
Historically, Google’s primary function was crawling and indexing web pages based on hyperlinks. This method helped search engines determine which pages were authoritative based on their backlink profile. However, search has evolved, and Google now focuses on crawling entities and mapping their relationships.
For example, when analyzing a sports article about Bryce Harper, Google recognizes that he plays baseball for the Philadelphia Phillies. The search engine assigns confidence scores to associations between entities, such as “Bryce Harper plays for Philadelphia Phillies.” These associations help Google refine its understanding of the subject matter, improving search result relevance.
The role of Natural Language Processing (NLP) in search
Google employs Natural Language Processing (NLP) to extract and classify entities from content. NLP allows the search engine to:
- Identify and categorize nouns, especially proper nouns like people’s names, business names, and locations.
- Extract attributes and properties of entities (e.g., “Bryce Harper is an outfielder”).
- Measure the strength of association between entities based on historical and real-time data.
This technology enables Google to build a robust Knowledge Graph that powers many search features, including featured snippets, People Also Ask (PAA) sections, and Knowledge Panels.
Schema markup: A tool for explicit entity recognition
One of the most effective ways to help Google recognize entities on a webpage is through schema markup. Schema markup provides structured data that tells search engines what a page is about. While Google’s documentation acknowledges the importance of schema for rich results, tests conducted by Dixon Jones and InLinks suggest that certain schema types can influence rankings beyond just structured data enhancements.
For instance, topic-based schema helps search engines categorize content more effectively. By labeling key topics and their relationships, schema markup aids in disambiguating entities and reinforcing context.
The impact of Google’s Knowledge Graph
Google’s Knowledge Graph is an extensive database that stores relationships between entities. It allows Google to surface information dynamically, whether in carousels, featured snippets, or Knowledge Panels.
A prime example of this is Google’s ability to compile lists dynamically. If a user searches for “best science fiction novels of 2023,” Google generates a carousel based on entity extraction rather than merely retrieving a static webpage list. This illustrates how entity-based search impacts content visibility.
Entity-based internal linking for SEO
Dixon Jones emphasized the importance of entity-based internal linking, where pages within a site interlink based on entity relationships. This approach helps Google establish context across a website, reinforcing topical authority and improving rankings.
For example, if a website covers sports and has multiple articles about baseball players, linking between related entities (e.g., “Bryce Harper” to “Philadelphia Phillies”) strengthens the site’s thematic coherence. InLinks’ tool automates this process by suggesting relevant entity-based links.
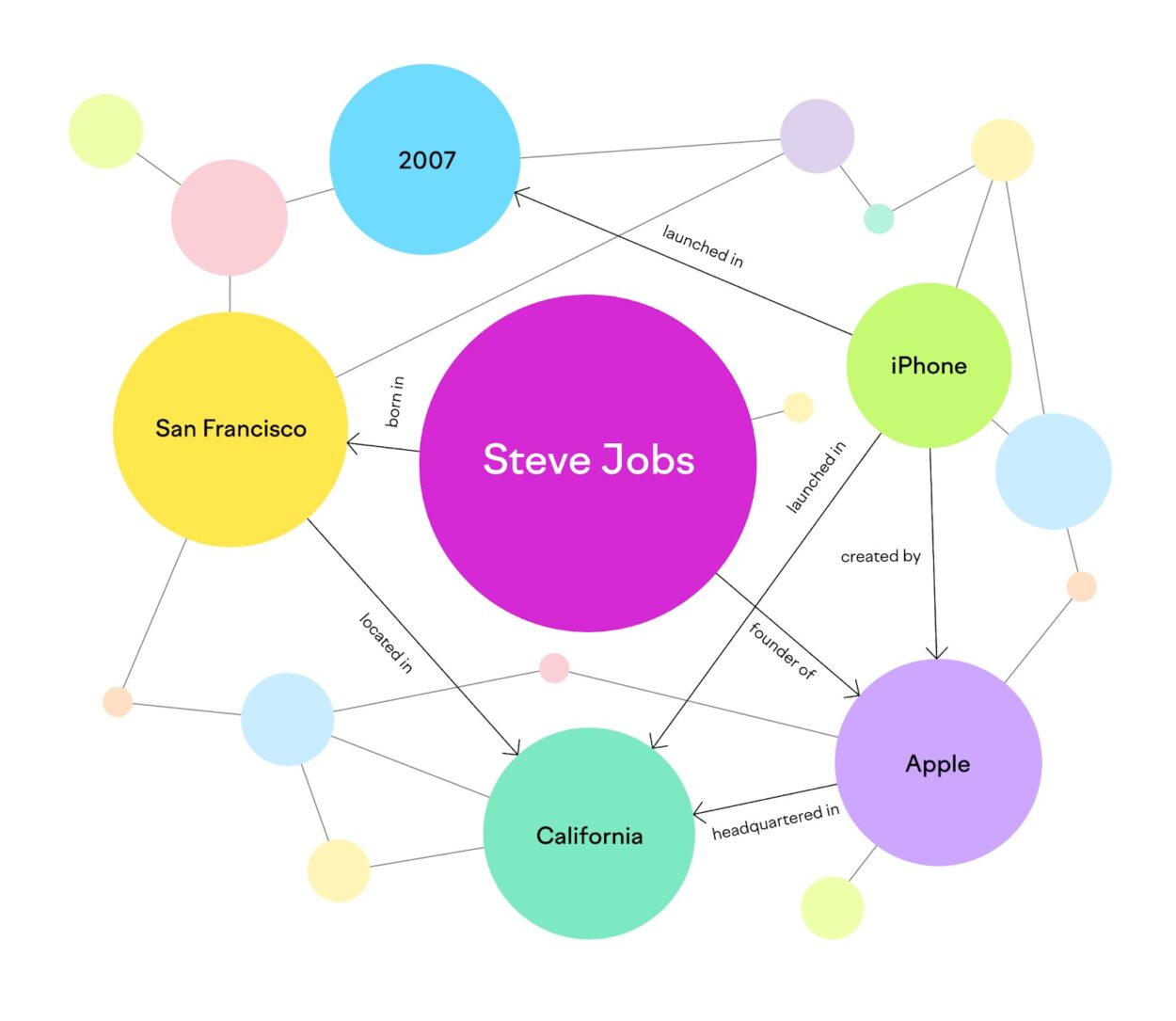
How Google handles information extraction
Google aims to deliver answers as efficiently as possible, reducing the need for users to visit multiple websites. This has led to zero-click searches, where Google provides direct answers in SERPs (Search Engine Results Pages).
For instance, searches like “What is my IP address?” or “currency conversion” yield direct answers without requiring users to click through to a website. This shift forces SEOs to rethink their strategies, focusing more on branding, engagement, and proprietary data that Google cannot easily replicate.
Challenges and considerations for SEO professionals
- Fact-checking and accuracy: Google assigns confidence scores to entity relationships, but errors can occur. Businesses and individuals must actively monitor their Knowledge Panel and online presence to ensure accuracy.
- Shifting business models: As Google answers more queries directly, websites relying on simplistic informational content must innovate. Thought leadership, proprietary research, and interactive experiences are becoming key differentiators.
- Optimizing for multiple search features: Beyond traditional rankings, SEOs should consider optimizing for carousels, voice search, and Google Discover, which rely heavily on entity-based search.
The future of SEO and AI-driven search
With advancements in AI, search engines will continue refining their ability to understand context and intent. Features such as:
- Google Discover: Uses entity-based relationships to suggest content to users based on browsing behavior.
- Voice search optimization: Conversational queries require more profound entity recognition, as users expect spoken answers rather than lists of links.
- Image and video recognition: Google increasingly analyzes multimedia to extract entity data, enhancing search relevance.
Dixon Jones concluded that embracing entity-based SEO is no longer optional. Brands must ensure their digital presence is well-structured, contextually relevant, and aligned with how search engines interpret information.
Final words
The transition from keyword-based SEO to entity-based search marks a fundamental shift in how search engines process and rank content. Google’s reliance on the Knowledge Graph, NLP, and schema markup means that SEOs must prioritize context, relationships, and structured data to maintain visibility.
Understanding and implementing semantic SEO strategies will be crucial for businesses aiming to stay ahead in an AI-driven search landscape. By leveraging entity recognition, schema markup, and strategic internal linking, websites can enhance their relevance, authority, and long-term sustainability in search results.
Follow us on X, Facebook, or Pinterest