As artificial intelligence (AI) reaches the peak of its popularity, researchers have warned the industry might be running out of training data — the fuel that runs powerful AI systems. This could slow down the growth of AI models, especially large language models, and may even alter the trajectory of the AI revolution.
But why is a potential lack of data an issue, considering how much there is on the web? And is there a way to address the risk?
Why high-quality data are important for AI
We need a lot of information to train powerful, accurate, and high-quality AI algorithms. For instance, ChatGPT was trained on 570 gigabytes of text data, or about 300 billion words.
Similarly, the stable diffusion algorithm (which is behind many AI image-generating apps such as DALL-E, Lensa, and Midjourney) was trained on the LIAON-5B dataset comprising 5.8 billion image-text pairs. If an algorithm is trained on an insufficient amount of information, it will produce inaccurate or low-quality outputs.
The quality of the training information is also important. Low-quality data, such as social media posts or blurry photographs, are easy to source, but aren’t sufficient to train high-performing AI models.
Text taken from social media platforms might be biased or prejudiced, or may include disinformation or illegal content that could be replicated by the model. For example, when Microsoft tried to train its AI bot using Twitter content, it learned to produce racist and misogynistic outputs.
This is why AI developers seek out high-quality content, such as text from books, online articles, scientific papers, Wikipedia, and certain filtered web content. The Google Assistant was trained on 11,000 romance novels taken from the self-publishing site Smashwords to make it more conversational.
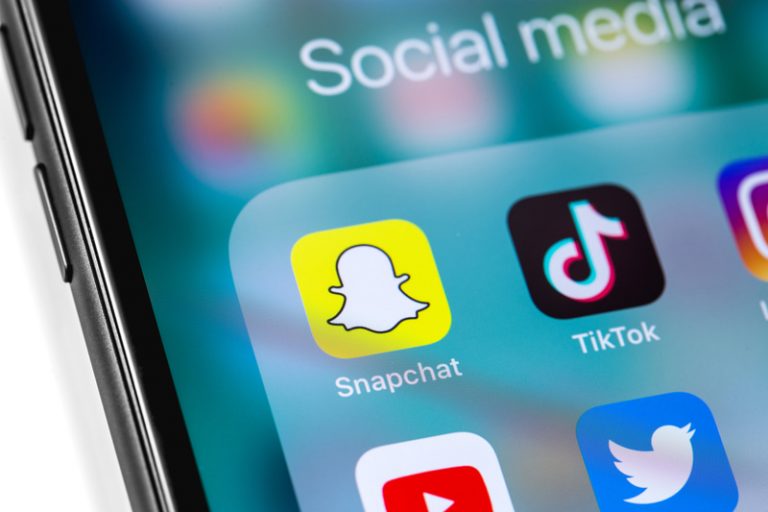
Do we have enough data?
The AI industry has been training AI systems on ever-larger datasets, which is why we now have high-performing models such as ChatGPT or DALL-E 3. At the same time, research shows online information stocks are growing much slower than datasets used to train AI.
In a paper published last year, a group of researchers predicted we will run out of high-quality text data before 2026 if the current AI training trends continue. They also estimated low-quality language data will be exhausted sometime between 2030 and 2050 and low-quality image data between 2030 and 2060.
AI could contribute up to US$15.7 trillion (A$24.1 trillion) to the world economy by 2030, according to accounting and consulting group PwC. However, running out of usable information could slow down its development.
Should we be worried?
While the above points might alarm some AI fans, the situation may not be as bad as it seems. There are many unknowns about how AI models will develop in the future, as well as a few ways to address the risk of data shortages.
One opportunity is for AI developers to improve algorithms so they can use the information they already have more efficiently.
It’s likely in the coming years that they will be able to train high-performing AI systems using less data and possibly less computational power. This would also help reduce AI’s carbon footprint.
Another option is to use AI to create synthetic data to train systems. In other words, developers can simply generate the information they need, curated to suit their particular AI model.
Several projects are already using synthetic content, often sourced from data-generating services such as Mostly AI. This will become more common in the future.
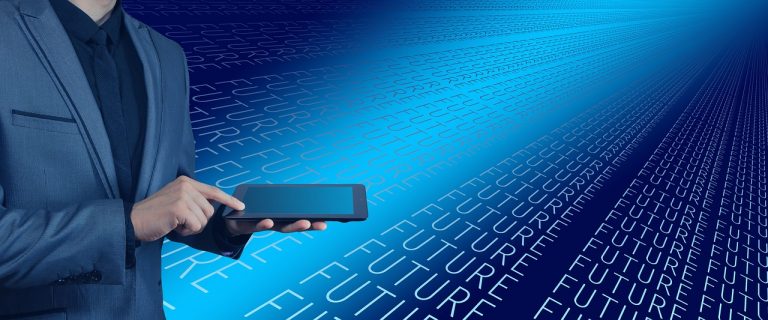
Developers are also searching for content outside the free online space, such as that held by large publishers and offline repositories. Think about the millions of texts published before the Internet. Made available digitally, they could provide a new source of data for AI projects.
News Corp, one of the world’s largest news content owners (which has much of its content behind a paywall), recently said it was negotiating content deals with AI developers. Such deals would force AI companies to pay for training data — whereas they have mostly scraped it off the internet for free so far.
Content creators have protested against the unauthorized use of their content to train AI models, with some suing companies such as Microsoft, OpenAI, and Stability AI. Being remunerated for their work may help restore some of the power imbalance that exists between creatives and AI companies.
Rita Matulionyte, Senior Lecturer in Law, Macquarie University
This article is republished from The Conversation under a Creative Commons license. Read the original article.
Follow us on X, Facebook, or Pinterest